Our research areas
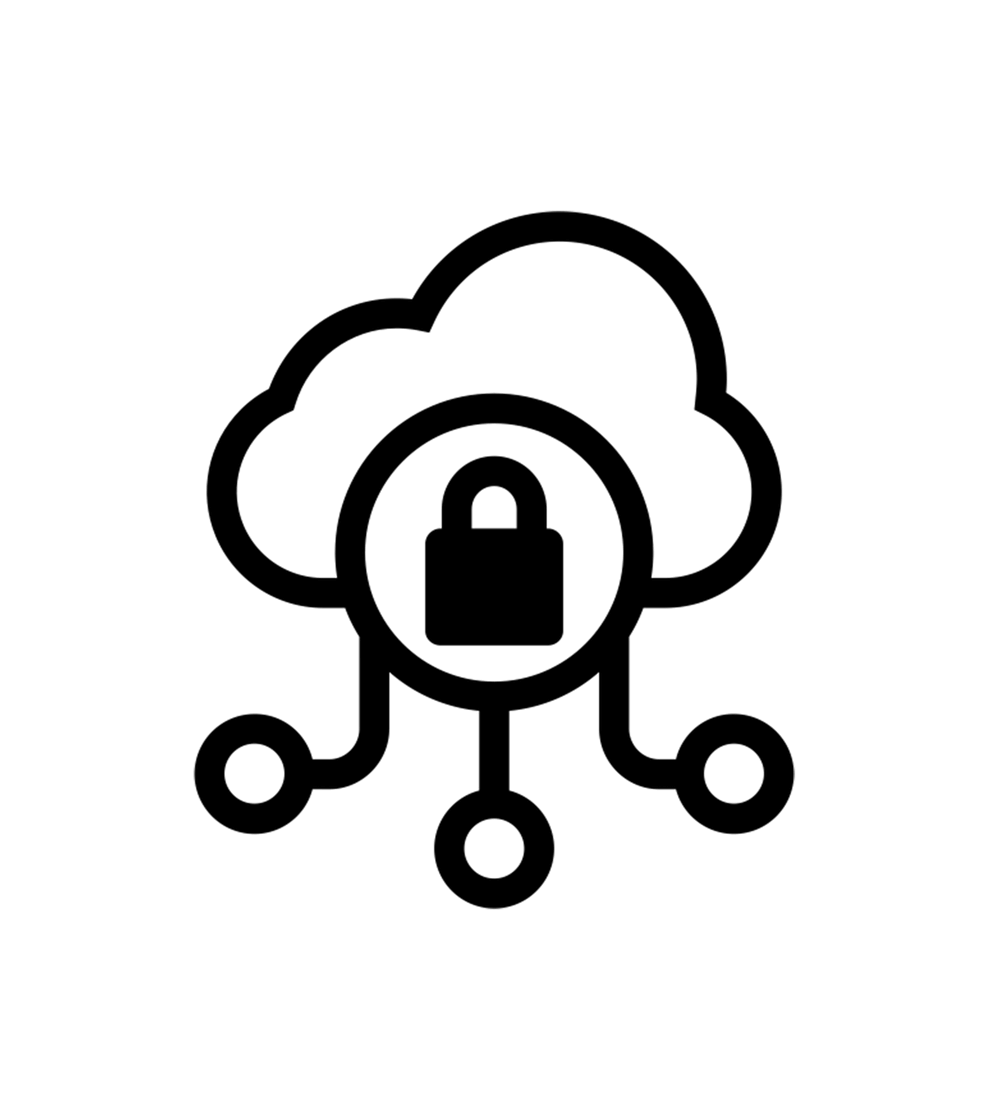
Secure and Robust Collaborative Learning
Machine learning algorithms continue to achieve remarkable success in a wide range of applications. These advancements are possible, in part, due to the availability of large domain-specific datasets, for training machine learning models. Hence, there are expanding efforts to collect more representative data to train models for new applications. This raises serious concerns regarding the privacy and security of the collected data. The privacy ramifications of massive data collection in the machine learning landscape have led both industry and academia to work on alternative privacy preserving paradigms of machine learning. Decentralized, secure machine learning have emerged as a compelling alternative for sensitive applications in the last few years. These paradigms eliminate the need to pool data centrally for ML training and thus ensure data sovereignty and alleviate the risks... Continue reading
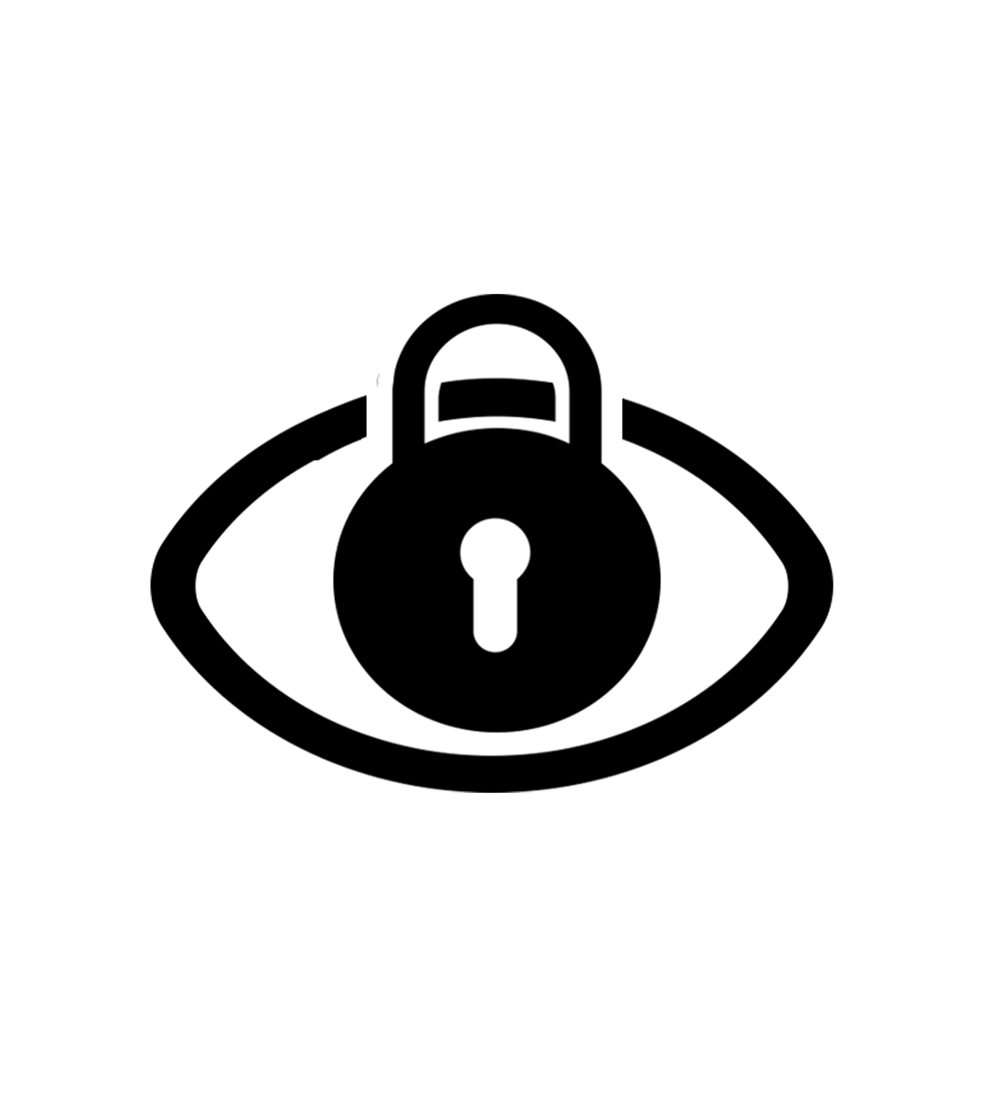
End-to-End Designs for Data Privacy
As increasing quantities of sensitive data are collected to gain valuable insights, the need to natively integrate privacy controls in data frameworks is growing in importance. Today, existing data protection systems are focused on ensuring that access to data is limited to authorized services, using security controls such as access control and encryption. However, once such a service is authorized to access the data, they have an unrestricted view of the data, which accounts for much of the data misuse today. We ultimately need to ensure that users' privacy preferences are respected even by authorized services. Privacy solutions that control the extent of what can be inferred (i.e., data minimization and purpose limitation) from data and protect individuals' privacy (i.e., differential privacy) are crucial if we are to continue to extract utility from data safely.In this project, we... Continue reading
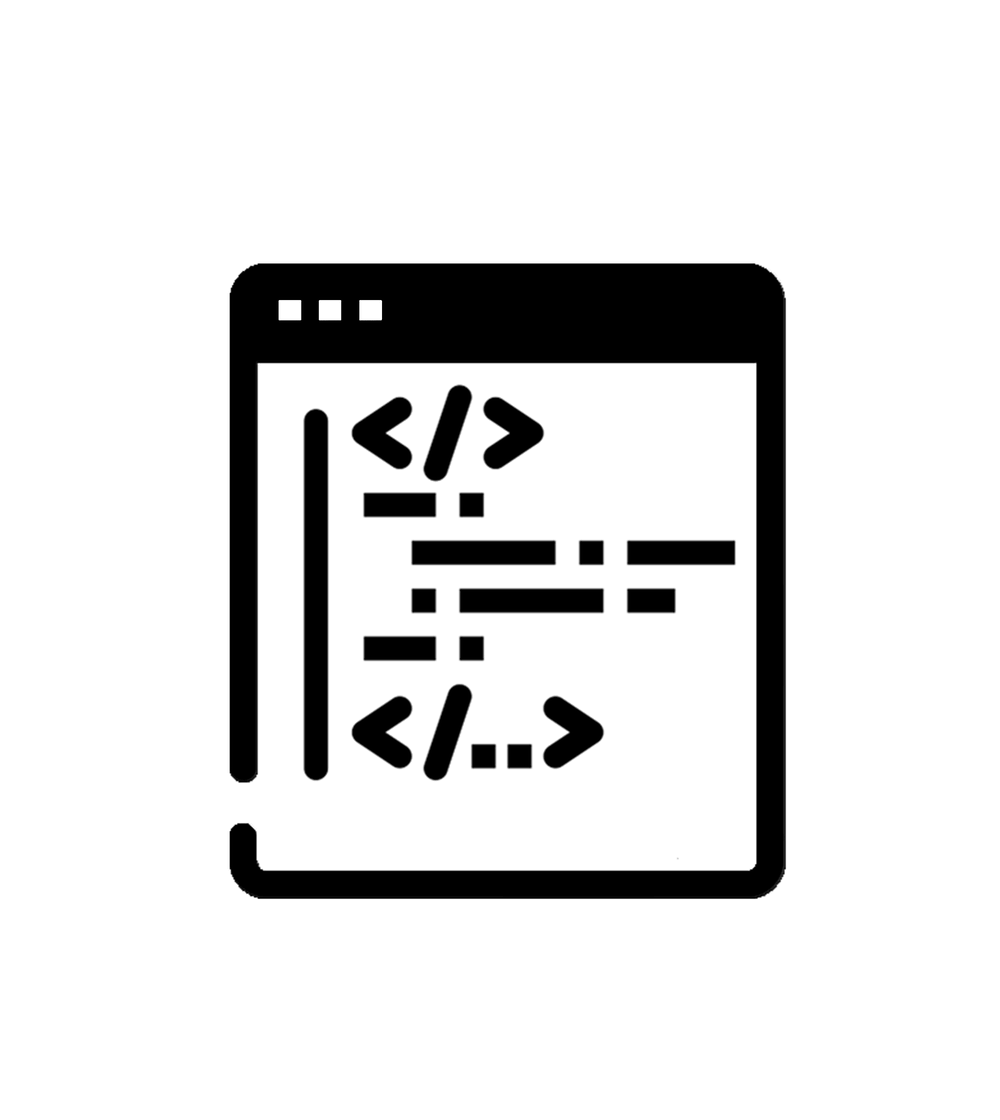
Accessible Privacy Preserving Computation
Privacy and security are gaining tremendous importance across all organizations as public perception of these issues has shifted and expectations, including regulatory demands, have increased. This, in turn, has led organizations to adopt stronger security and privacy protection. Although industry best practice such as in-transit and at-rest encryption provide important protection for user data, the need to decrypt data to compute on it (in-use) still exposes the data to a wide variety of threats. Secure computation techniques such as fully homomorphic encryption (FHE), which allows a third party to perform arbitrary computations on encrypted data, address this gap. In recent years, we have seen a leap in performance in FHE driven by a series of breakthroughs and advancements that have propelled FHE into the realm of practical applications. With hardware accelerators on the horizon,... Continue reading
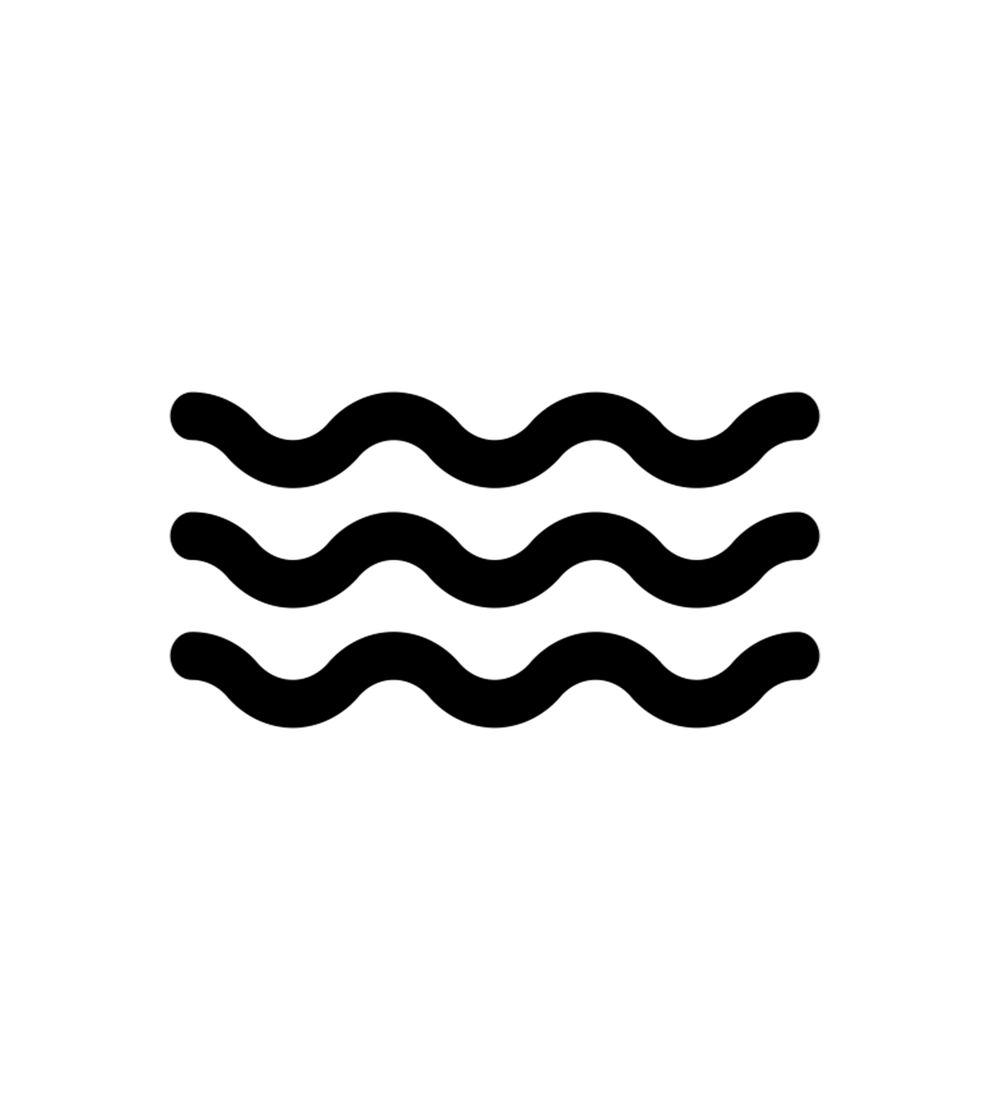
Privacy Preserving Stream Analytics at Scale
Recent years have seen unprecedented growth in networked devices and services that continuously collect increasingly detailed information about individuals. The collection of this unbounded stream of data is increasingly prevalent across a wide range of systems in diverse domains such as health, agriculture, transportation, operational insight, and smart cities. The growth of streaming data is largely attributed to the rising demand for instrumentation. Individuals and organizations are continuously logging various metrics that report systems’ state for better diagnoses, forecasting, decision making, and resource allocation. However, with this trend comes the problem of ensuring the privacy of user data. Users today typically entrust their data to a thirdparty storage or application provider. However, there is growing concern that this model leaves users vulnerable to privacy... Continue reading